HOME >> Single Blog
What is RFM Model & RFM Analysis?
Published: January 26, 2023 | null MIN READ
The most classic analysis model of user behavior analysis is the RFM model, which is simple, easy to operate, and highly practical. In this article, we will introduce in detail what the RFM model is, how to analyze the RFM model, and how to quickly build an RFM model for data analysis.
The RFM model is an important tool and method for measuring customer value and profitability. Analyzing the RFM model is a crucial part of user behavior analysis.
How to use data analysis to help business growth is a question that every data analyst considers. In recent years, there has been a lot of talk about precision operations, data-driven growth, and growth hackers. The core behind these concepts is user behavior analysis.
The most classic analysis model of user behavior analysis is the RFM model, which is simple, easy to operate, and highly practical. In this article, we will introduce in detail what the RFM model is, how to analyze the RFM model, and how to quickly build an RFM model for data analysis by using a professional BI tool.
What is the RFM Model?
The RFM model is an important tool and method for measuring customer value and profitability, which evaluates a customer's value to a company based on three indicators: Recency, Frequency, and Monetary Value.
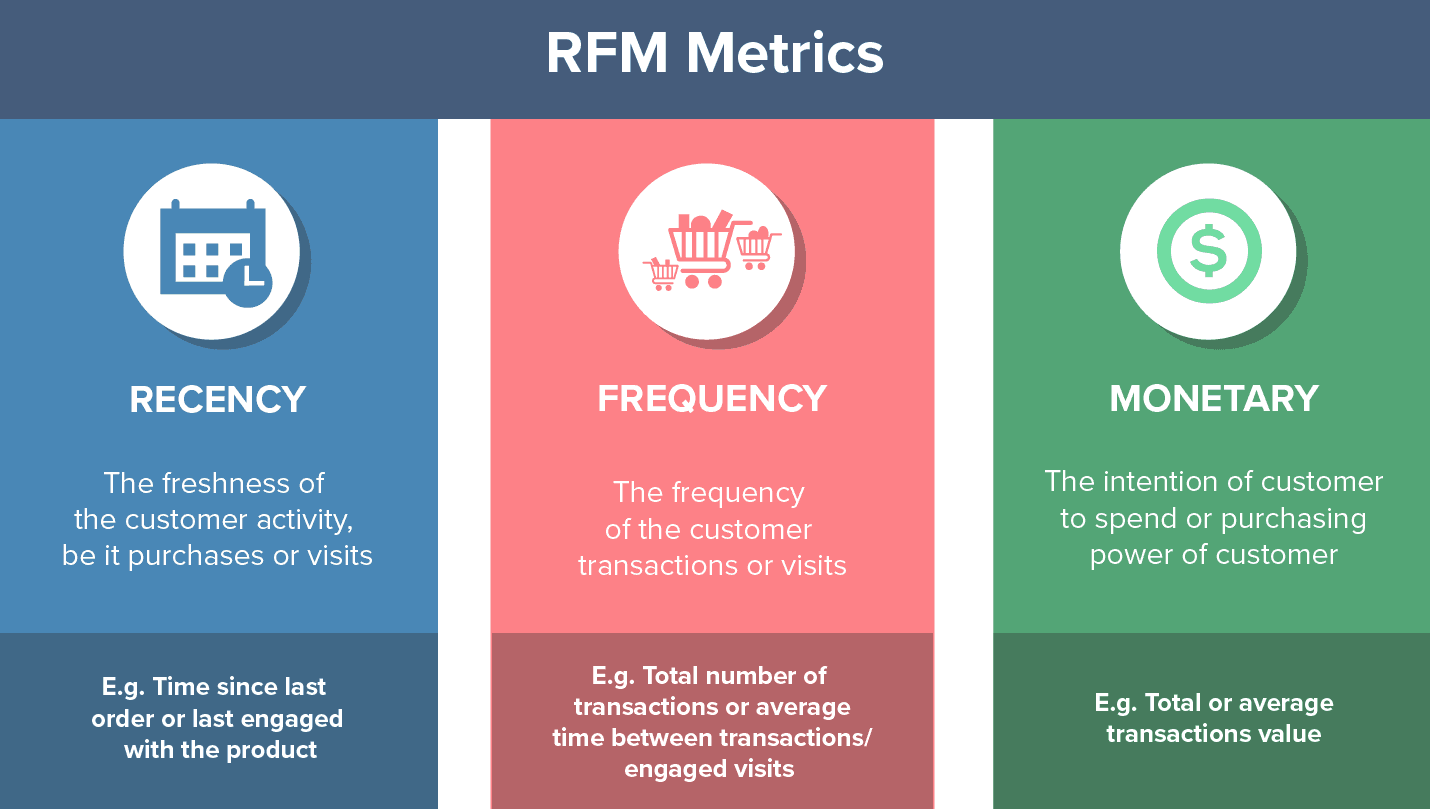
- "R" (Recency) refers to the time since a customer's last purchase, indicating the length of time since the customer's last order was placed, which is directly related to customer churn and repurchasing.
- "F" (Frequency) refers to a customer's frequency of purchase, or the number of times a customer has made purchases within a fixed period. This indicator reflects a customer's purchase activity, with customers who purchase more frequently being more likely to be loyal to the brand.
- "M" (Monetary Value) refers to the amount of money a customer spends, or how much a customer spends on the platform during a fixed period, which directly reflects the customer's contribution value to the company.
How to Do RFM Analysis?
According to the level of RFM indicators, eight types of customers with different value attributes to the company can be distinguished. Each type of customer requires different marketing methods to operate. The following are the characteristics of these eight types of customers and the corresponding marketing strategies.
Key Valuable Customer (R↑, F↑, M↑)
Key valuable customers are customers with high repurchase rates, high purchase frequency, and high monetary value. They are the most valuable users of a company. Companies need to maintain relationships with Key valuable customers constantly and use rich marketing methods to continuously improve their brand stickiness and loyalty.
Key Potential Customer (M↑, R↑, F↓)
Key potential customers refer to customers who have recently made purchases, and have spent a relatively large amount of money but do not make frequent purchases. They are essential customers for a company. For these customers, companies need to continue to motivate them through refined marketing strategies, increase their frequency of purchase, and develop them into key valuable customers.
Key Loyal Customer (F↑, M↑, R↓)
Key loyal customers are customers who have a high purchase frequency, and high monetary value but have not made any purchases recently. For this type of customer, it is recommended that the company actively understand the recent changes in customer needs through questionnaires, consultations, and gift feedback and formulate corresponding solutions to prevent the loss of this type of customer.
Key At Risk Customer (M↑, R↓, F↓)
Key at-risk customers are customers who have spent a relatively large amount of money but have low purchase frequency and have not made any purchases recently. This type of customer can consume and can bring value to the company. The company should actively understand the needs of this type of customer, understand the reasons why they have not made any purchase in such a long period, and then formulate solutions to prevent the loss of this type of customer.
General Valuable Customers (R↑, F↑, M↓)
General valuable customers refer to customers with high repurchase rates, and high purchase frequency, but small monetary value. This type of customer has less potential monetary value but is very active and has higher brand loyalty. This part of customers can be used to promote brand activities and expand brand influence and reputation.
General Potential Customers (R↑, F↓, M↓)
General potential customers refer to customers who have recently made purchases but have relatively low purchase frequency and have spent only a little money. These customers may be trying to make their first purchase and are still in the brand recognition stage. Companies can use brand marketing activities for the continuous cultivation of this type of customer.
General Loyal Customers (F↑, R↓, M↓)
General loyal customers refer to customers with a relatively high purchase frequency, but low consumption amount, and have not made any purchases recently. This type of customer has a certain degree of brand loyalty but may have recently had changes in their needs. Companies can use brand marketing activities to awaken their interest.
General At Risk Customers (F↓, R↓, M↓)
General at risk customers have low values in all three RFM indicators. These customers have a low level of activity, and it is difficult to maintain their loyalty to the brand. Companies can use targeted marketing strategies, such as product promotions or customized services, to retain these customers.
How to Quickly Build RFM Model for RFM Analysis?
The RFM model can provide a reasonable estimate of a customer's lifetime value by measuring the level of customer value based on an ideal customer profile. Through such analysis, it is possible to identify the group that is most likely to become loyal customers of the brand and allow companies to focus their efforts on the most valuable customers.
Next, we will directly begin the practical tutorial for RFM model analysis, without the need to write code, which is very convenient!
We will use a sales dataset as the data source and perform a simple analysis of user behavior. In summary, there are several steps:
1. Customer Segmentation
The first step in conducting customer behavior analysis is to classify your customer groups according to the actual business needs of your company, which is essential for effective demand shaping. The attributes to consider for customer segmentation mainly fall into three categories:
- Basic attributes: such as gender, age, and location;
- Interest attributes: such as media preferences and transaction behavior;
- Custom attributes: attributes defined based on industry characteristics, such as whether a person has children, which is important for the dairy industry.
2. Define Metrics
Defining metrics involves calculating RFM (Recency, Frequency, Monetary) metrics for each customer using tools like Excel or BI software. In this context, FineBI, a leading self-service BI tool from FanRuan, is utilized to analyze data on each customer's last purchase time (R), purchase frequency (F), and purchase amount (M) based on their name, transaction time, and transaction amount.
FineBI is an advanced big data analytics and business intelligence solution designed to meet diverse data analysis needs. Its user-friendly interface enables users of all skill levels to effortlessly create professional BI dashboards, conduct self-service data analysis, and derive actionable insights, ultimately empowering businesses to make data-driven decisions and drive growth.
Through simple operations like drag-and-drop, you can create professional dashboards without difficulty!
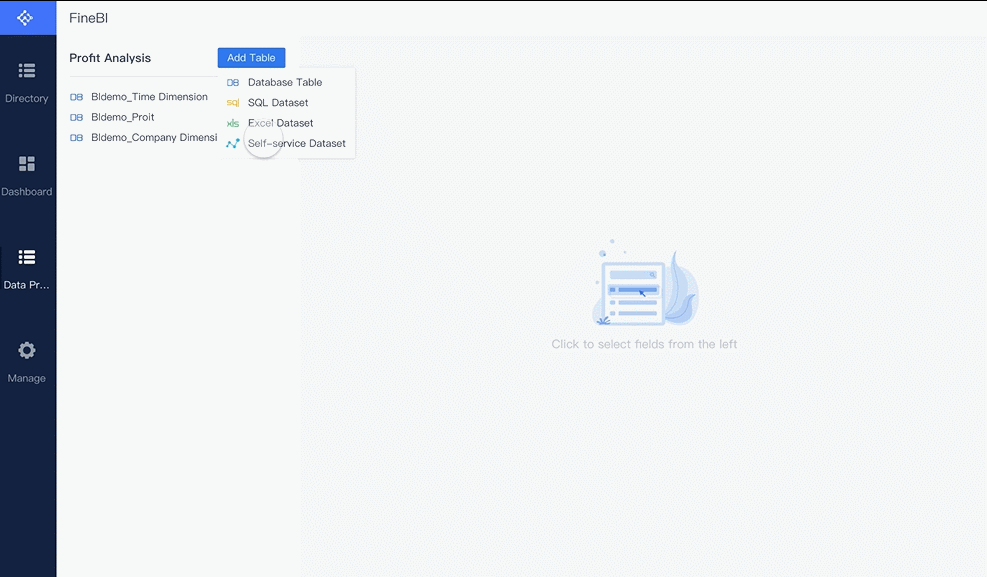
Here is a tip for defining metrics: it is important to note that these three indicators are not rigid and unchangeable. Remember to be flexible and adaptable based on the characteristics of your own industry. For example, in the financial industry, the most recent purchase time may not be applicable, so it may be more appropriate to use the length of time that a customer holds a financial product instead of the last transaction interval, which better reflects the length of time a user has established a connection with a financial company. A unity dashboard can be a valuable tool in this context, allowing financial institutions to easily customize and track these dynamic metrics, providing real-time insights that are relevant to both the organization and its customers.
3. Data Connection
FineBI supports various types of data connection methods, such as connecting through databases like Oracle, SQL, etc. Here, I directly imported an Excel table into FineBI, which can easily handle non-real-time data.
However, if we have data from multiple data sources and need to select different indicators from each to integrate and analyze, what can we do?
Here, FineBI has a very user-friendly advantage, which is the ability to integrate data indicators through self-service data sets. As shown in the figure below, we can directly select "New Self-Service Dataset", choose the table, and add fields. We can find any required indicators from the tables, which is very convenient.
4. Data Calculation
Then calculate the consumption indicator for each customer.
There are three key indicators for each customer:
- Recency per customer (R)
- Frequency of consumption per customer (F)
- Montary(M): Calculate the average of the total consumption amount of the customer before calculating the average consumption amount of each user.
In FineBI, data processing in business reports is very convenient. Through functions such as filtering and merging calculations in FineBI, data can be processed quickly without writing SQL statements or other codes.
Through simple operations like drag-and-drop, you can create professional dashboards without difficulty.
Firstly, we should calculate the average consumption.
1) Click "+" and select "New column", as shown below:
2) Name the newly added column "Average Consumption", select "all values/within group" to set it, and click "OK" to obtain the overall average indicator "Average Consumption". As shown below:
Detailed Settings of "all Values/within group" :
- Value rule: all values
- Value from: "MONEY"
- Statistical method: "Average"
Then, we can calculate the average consumption (M) and consumption frequency (F).
1) Click "+" and select "Group Summary", as shown below:
2) Drag the field on the left to the corresponding group summary box on the right, and set the summary indicator to display the results to complete the sorting of the original data. As shown below:
Summary of indicator settings in the box:
- DATE: Latest time
- "MONEY" : "Average"
- "AMOUNT" : “Records Count”
The groupings obtained in the "Data Preview" section are summarized to obtain key indicators of customer characteristics. The "MONEY" and "AMOUNT" fields respectively represent Average Consumption (M) and Consumption Frequency (F).
At the next step, let's calculate the last consumption distance time (R).
Click "+" and select "New column", as shown below:
Set the new column name as "Last consumption distance Time", select "time difference" and click "OK" to obtain "Last consumption distance time (R)", as shown below:
Setting details of "time difference" :
- Time difference = "Current Date" - "DATE"
- Measurement method: “day”
So far, three customer key indicators and an overall average value are obtained in this section: "Last consumption distance time" (R), "AMOUNT" (F), "MONEY" (M) and "Average Consumption".
Then, we should calculate their corresponding overall average values.
- Calculate the average consumption frequency:
1) Click "+" and select "New column", as shown below:
2) Set the name of the new column to "Average consumption frequency", select "all values/within group", and click "OK" to obtain the overall average value indicator "Average consumption frequency". As shown below:
Setting details of "all values/within group" :
- Value rule: all values
- Value from: "AMOUNT" (F)
- Statistical method: "Average"
- Calculate the average time of the last consumption distance
1) Click "+" and select "New column", as shown below:
2) Set the name of the new column as "Average time of last consumption distance", select "all values/within the group", and click "OK". The overall mean indicator "Average time of last consumption distance" was obtained. As shown below:
Setting details of "all values/within group" :
- Value rule: all values
- Value from: "Last consumption distance time" (R)
- Statistical method: “Average”
The three key indicators obtained and their corresponding overall average values are shown in the figure below:
5. Vectorization of customer characteristics
Vectorization of customer characteristics based on whether key indicators are greater than the overall average level of customers.
Where, in IF(XXX > XXX average value,1,0), the value less than the population average is set to "0", and the value greater than the population average is set to "1", so that "1" and "0" maintain positive characteristics and negative characteristics.
- Amount vectorization
Click "+" and select "New column", as shown below:
Name the new column "Amount vectorization", enter the formula IF(MONEY>Average Consumption, 1,0) and click "OK", as shown below:
- Frequency vectorization
Similarly, add the "Frequency vectorization" field, as shown below:
- Last consumption vectorization
Similarly, the field of "Last consumption vectorization" is calculated. When entering the formula, it should be noted that "Last consumption distance time" < "Average time of last consumption distance" belongs to the positive vector, representing "1". As shown below:
6. User Classification
The characteristic vector values of customers have been obtained, and customers can be classified according to the following table:
Customer characteristics | Customer classification |
Key valuable customer (111) | Recent purchase time, purchase frequency and monetary value are all high (VIP) |
Key potential customer (101) | Recent purchase time is relatively recent, monetary value is high, but frequency is not high, which means the customer's loyalty is not high, but they are very potential users, so the company must focus on cultivating this type of customers. |
Key loyal customer (011) | Recent purchase time is far away, but the money spent and frequency are very high. |
Key at risk customer (001) | The customers' recent purchase time is far away and the frequency of purchase is low, but the money they spent is quite a number. They are may be losing or already losing users to the company, measures should be taken to retain these customer. |
General valuable customer (110) | The recent purchase time is close, the frequency is high but the monetary value is low, so average transaction value of the customer needs to be increased. |
General potential customer (100) | Recent purchase time is close, monetary value, frequency is not high. |
General loyal customer (010) | Recent purchase time is far away, purchase frequency is high, but the amount is not high. |
General at risk customer (000) | Recent purchase time, purchase frequency and monetary value are all not high. |
- Add RFM indicator
Click "New column" and use CONCATENATE() to CONCATENATE RFM vectorization values, as shown below:
- RFM custom grouping
1) Add "Group Summary", as shown below:
2) Drag the following fields into the group box and set the display value of "DATE" to "Latest time". As shown below:
3) Set the RFM field to "Custom Grouping", as shown below:
4) Grouping is based on the customer type analysis table in section 2.5.1. Click "011" "Add a group" and name the group, and then click the next ungrouped indicator to add a group, as shown below:
5) Click "OK", then click "Save" to save the self-service dataset. As shown below:
Click the created "RFM Data Analysis" for "Data Preview" to view the Data. As shown below:
At this point, the self-service dataset can also be visualized through the dashboard of customer categorization-related data.
7. Data Visualization
By using FineBI to segment customers, it can be turned into a data visualization analysis dashboard in FineBI for customer analysis as needed.
FineBI supports more than 50 chart styles, covering all basic and high-level charts on the market, and also has excellent dynamic effects and a powerful interactive experience. Various features can be set according to the needs during use, and can also be self-adjusted and displayed on the mobile terminal and large LED screen.
The effect of the dashboard is shown in the figure below:
Use the pie chart and table at the top for analysis:
- The majority of customer types are general development customers (recently purchased, but with low frequency and amount), and the company's main business should be promoted to these customers through advertising and promotion to deliver product information to their hands.
- The second largest proportion is general retention customers (who have not made a purchase for a long time and have lower frequency and amount of purchases). Promotional activities should be launched for this group to stimulate consumption.
Use the bubble chart at the bottom for analysis:
- The bubble chart uses M and F as the horizontal and vertical axes to represent the customer's spending ability, with larger values indicating higher spending ability. R is a negative quota, which represents the consumption loss situation. The larger the graph, the longer the recent consumption time, which reflects the condition of customer loss.
- The bubble chart shows the customer consumption ability and the situation of customer loss in various regions. The analysis shows that customers in Chengdu and Beijing have higher consumption amounts, but more serious customer loss situation, which requires special attention.
- Customers in Wuhan and Shenyang mainly have small amounts of purchase behavior, but a higher frequency of purchases.
The RFM model is widely used in user behavior analysis. Due to time constraints, this is just a simple tutorial. FineBI also has more related data and data models for everyone to practice! Using FineBI makes it easy to build various classic data analysis models, such as the KANO analysis model discussed in this article, as well as other models like the BCG Matrix (Growth Share Matrix), Kano Model Analysis, DuPont Analysis Model, etc., to help businesses gain insights.
FineBI also offers business theme analysis scenarios for different industries, including manufacturing, pharmaceuticals, retail, finance, and more. By analyzing and displaying business indicator data, relevant managers can easily grasp business dynamics.
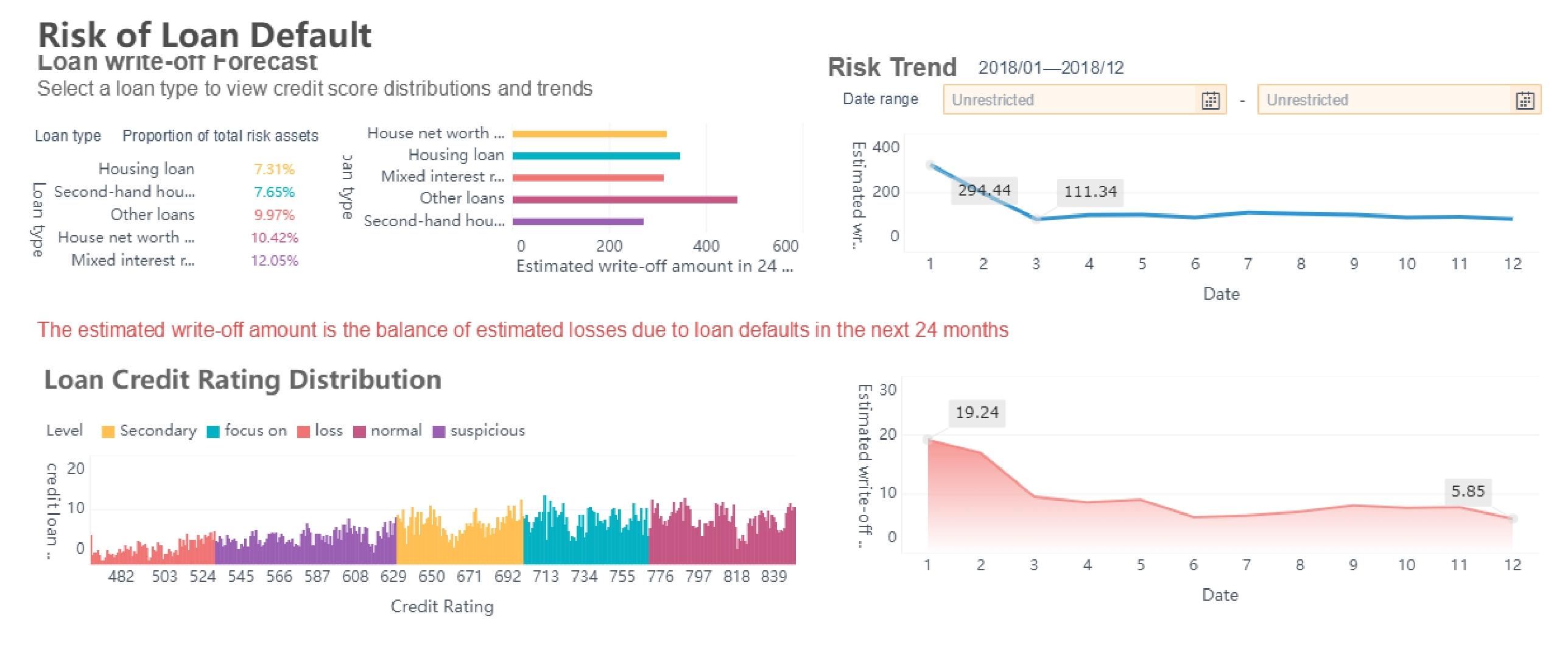
FineBI can liberate business professionals from the quagmire of data processing and visualization, allowing them to focus more on data analysis, management, and business communication.
FineBI is free for personal use. For enterprises, it offers a quote-based plan that charges according to different situations. In a word, FineBI is price-friendly to all customers.
Click the button below to try FineBI for free. Give it a try today!
Related Article
who read this article also viewed
2024-08-09 By Lewis
What is Descriptive Analytics?
Understand descriptive analytics, its role in data analytics, and how it uses historical data to identify trends and improve decision-making.
2024-08-08 By FineBI
Six Essential Steps in the Data Analytics Process
Master the six essential steps in the data analytics process: Ask, Prepare, Process, Analyze, Share, and Act. Enhance your data-driven decision-making.
2024-08-08 By Lewis
What is Enterprise Data Analytics and How Does it Work?
Understand Enterprise Data Analytics, its key concepts, types, tools, and practical applications. Learn how it improves decision-making and operational efficiency.
Start a new journey of business intelligence and big data analysis with FineBI
Try it now and get over 100 data analysis templates for business scenarios in various industries.
Try FineBI for Free